Knowm’s Notes
Despite many folks like myself theorizing over the last decade about memristors and the circuits that can be built from them, it is important to understand that we are only just now, as a global community, actually learning how to use them. As the first company to offer a commercial discrete memristor, we at Knowm understand the need for universal performance measures and we take note when somebody gets closer to ‘cracking the memristor code’, so to speak. On that note, it’s time for a lesson. A collaboration of researchers from Chile and Spain recently published some work that everybody should see (free download until Feb 9, 2020):
The paper is concerned with the consequential problem of understanding how to accurately drive incremental conductance changes in memristors and looks at two memristor devices: A W+SDC Knowm Memristor and a custom fabricated HfO2 device [TiN(200)/Ti(10)/HfO2(10)/W(200) nm]. The group took careful measurements of conductance change and related this to the accumulated energy applied to the devices over time. The results show switching energy is insensitive to the biasing conditions and that “resistance change per energy unit” is a performance parameter for characterizing memristor technology. To quote the authors:
Our experimental results…confirm that the energy, and particularly the “resistance change per energy unit” (dR/dE), is a comprehensive magnitude, suitable not only for the behavioral analysis of the analog operation of memristors, but also to be used as a universal performance parameter capable of characterizing quantitatively different RS device families.
While I highly recommend you read the paper for yourself, here is my understanding of their findings.
The energy to drive the memristor into a low resistance state (“Set”) appears constant for all voltage rise times (VRS), while the energy to drive the memristor into a high resistance state (“Reset”) decreases as the VRS increases. The less resistive a memristor, the more energy it will dissipate in a set amount of time simply through normal resistive dissipation, just like any normal resistor. While we might desire the energy we apply to go into structural reconfigurations that alter resistance states, energy is simultaneously being lost to the conductive channel. If the channel starts off in a more conductive state, more energy will be lost before the structural reconfigurations lower the conductance.
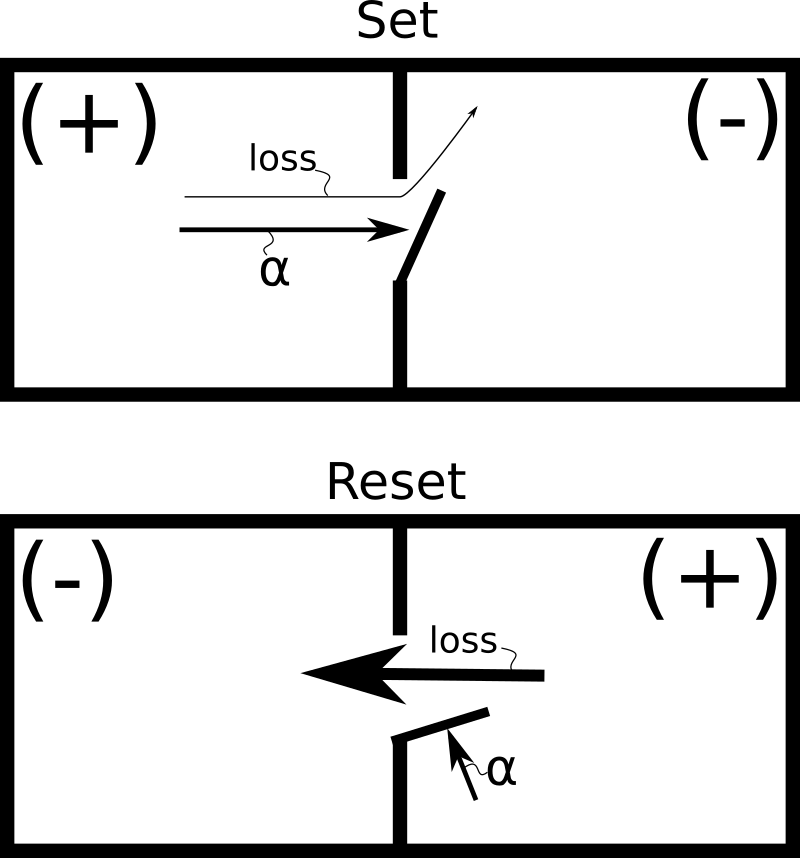
Energy lost to joule heating in memristor set and reset operations, as represented by a door joining two rooms. Unless the pressure between the rooms is very suddenly increased, significant energy will be lost in a reset operation.
Think of it like a door that conducts air between two rooms, as diagramed above. If we apply a pressure gradient across the rooms, air will move from one room (high pressure/+) to the other (low pressure/-). If the gradient is large enough and the door is initially open, the rushing air will slam the door shut quickly. Most of the energy will be directed to the door closing rather than equalization of pressure. On the other hand, if the pressure differential is built up slowly, most of the air will flow through the open door until the differential is large enough to swing the door closed. When it finally closes and we account for the energy lost we will find a large amount has simply dissipated through the open door (“Joule heating”) and a smaller amount was directed to moving the door (memristor reconfiguration or “α” in the paper). If the door is already closed and we apply a pressure gradient, it’s clear that much less air will escape around the door before it swings open, as all the energy is essentially directed to the door. By taking careful measurements the group was able to show how the energy lost to joule heating is minimized as the VRS increases. In the room analogy, if we want to close the door with high efficiency we need to cause a very sudden increase in the pressure differential above a threshold needed to move the door.
Beyond the findings of the paper, which are both clear and clearly-useful, I was pleased to see that the work was done with an Analog Discovery 2, a full-featured low-cost multi-function instrument which Tim and I discovered while developing Memristor Discovery. Disturbed by the high cost of almost all semiconductor equipment and the significant barrier it represents, we wanted to find a path where anybody could afford to do memristor science. This group not only used the AD2, but they designed a custom op-amp circuit PCB board for providing current compliance protection. Even better, they published this work open-access!
Leon Chua famously said “If its pinched its a memristor“, referring the characteristic I-V curve generated from a periodic voltage applied to a memristor. I can think of no better initials for a man of memristor science than IV! I had the opportunity to ask the paper and memristor book co-author Ioannis Vourkas (IV) some questions, which I (Alex Nugent, AN) provide below.
Interview with paper co-author Ioannis Vourkas
[AN] How did you get into the memristor field? When did you first become aware of memristors in general and what about them attracted you to focus your research on them?
[IV] It was in late 2010, when I was starting my PhD studies at the Democritus University of Thrace (DUTh) in Greece, under the supervision of Prof. Georgios Sirakoulis. I remember in one of our first conversations hearing about memristors being a hot research topic, so it grabbed my attention. Back then, it was not easy to work in this topic, with almost nobody around at DUTh having previous experience so as to guide you properly in your first research steps. After a lot of reading, I realized the great potential of memristors to revolutionize electronics, being not just memory devices but instead a powerful tool in the hands of engineers! I was really excited to “walk on unexplored territory” and produce knowledge for such a promising emerging device technology. Moreover, it was really inspiring to me having the chance to meet Prof. Leon Chua in late 2012 during the ACRI2012 conference in Santorini, Greece. Ever since, I have focused my research efforts on memristor-based circuits and systems, mostly targeting in-memory and unconventional or neuromorphic computing applications.
many researchers all over the world working in memristor-related research topics, do not have access to real devices. In this context, the commercially available memristors released by Knowm Inc. actually brought this technology closer to all of us, the researchers that still do not have access to fabrication facilities.
[AN] What has been your overall experience with memristors so far?
[IV] I have done a great deal of work based only on simulations. Nevertheless, I believe that deeper understanding of memristive behavior is the only way towards maximum exploitation of the favorable properties and the analog nature of this new device technology in innovative applications; and this can be achieved through experimental hands-on experience with real devices. However, still many researchers all over the world working in memristor-related research topics, do not have access to real devices. In this context, the commercially available memristors released by Knowm Inc. actually brought this technology closer to all of us, the researchers that still do not have access to fabrication facilities. I was personally thrilled to be able to experiment with real devices. Apart from the chips in the new package that arrived this year, we still have and keep experimenting on the very first packaged BS-AF-W devices we got in late 2016! Having our own devices really opened us a wide range of opportunities, not only at device characterization level but also at circuit level. In my group here at the Electronic Engineering Department of Universidad Tecnica Federico Santa Maria in Valparaiso, Chile, as well as with my closest collaborators in Spain, USA, and Greece, we are currently working on the experimental validation of several circuit ideas previously seen functioning only in simulations.
[AN] Tell me about your measurement setup…
[IV] I have to admit that lab experiments with memristors, might look simple, but in fact constitute quite a challenging step, especially for the uninitiated. It took us a long time before we could feel really comfortable doing this. An important barrier in this direction is the lack of state-of-the-art equipment to realize reliable experimental measurements. Additionally, the parasitics and the still important memristor device variability can sometimes cause hesitation on the inexperienced researchers; not to mention the possibility to burn memristors accidentally. In this direction, the memristor discovery board coupled with the Digilent AD2 portable and programmable function generator & oscilloscope, was a safe way to start. We believe that measuring the memristor behavior accurately with low cost equipment will do more to promote memristor science around the world than almost anything else.
We believe that measuring the memristor behavior accurately with low cost equipment will do more to promote memristor science around the world than almost anything else.
In this context, we soon figured out a low cost setup composed of off-the-shelf components, which helped us gain valuable hands-on experience on memristor characterization. In such setup, we connect the memristor bottom electrode to the inverting input of an operational amplifier in a transimpedance amplifier topology. Such circuit enables: (i) controlling the voltage drop on the memristor terminals, (ii) sensing the current through it in a non-invasive manner via a current to voltage conversion, and (iii) adjusting the compliance current to protect the memristor from damage. It also allowed us to monitor the device resistance at all moments, computed as a function of the current and the voltage applied to the memristor. With this topology set up and calibrated, we were able to perform several types of measurements, most of which originated just by curiosity. It is really simple circuitry and given the ease of use of the Digilent AD2, anyone could start having their own data literally in no time. In this sense, we are confident that such ideas presented in a tutorial manner in [1] will help others to rapidly set up their own characterization topology and engage in real lab experiments.
[1] J. Gomez, I. Vourkas, and A. Abusleme, “Exploring Memristor Multi-Level Tuning Dependencies on the Applied Pulse Properties via a Low Cost Instrumentation Setup”, IEEE Access, vol. 7, pp. no. 1, pp. 59413 – 59421, 2019
[AN] What have you been able to conclude from your experience thus far with multi-level memrsitance tuning?
[IV] It is widely known to be challenging to program accurately and repeatedly the memristors to a specific desired state just by applying a single pulse at their terminals, owing to variability issues. Thus, several strategies, circuits and pulsing schemes have been proposed in the literature aiming to improve the controllability of multi-level resistive states of memristors.
In this sense, we suggested the memristor-resistor voltage divider circuit approach which we first validated theoretically exploiting the incremental threshold-type switching behavior of memristors (i.e. the fact that change-rate is significant above a voltage threshold) for rapid and accurate multi-state resistance tuning. In early 2019 we reported in [2] the experimental verification of this approach for accurate resistance tuning of memristors; thus, bridging the gap between theory and practice in this particularly interesting application. Indeed, the Knowm memristors can have their resistance controlled in an analog manner and they demonstrate a threshold-based behavior. So, the observed experimental results were in agreement with the previous simulation-based analysis. Moreover, the efficiency of this tuning approach was compared with a pulse-based precise resistance tuning protocol in terms of resistance tuning precision, average required tuning time, and average energy consumption, with very favorable results for our approach.
[2] J. Gomez, I. Vourkas, A. Abusleme, G. Ch. Sirakoulis, and A. Rubio, “Voltage Divider for Self-Limited Analog State Programing of Memristors”, (2019) IEEE Trans. Circ. Syst. ΙΙ: Exp. Briefs, in press
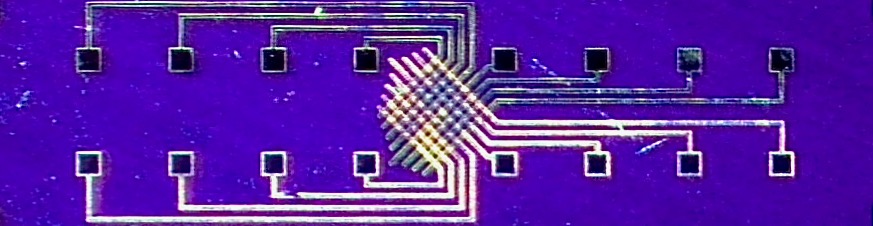
[AN] How would you summarize your recent work with the “Resistance Change per Energy Unit” paper?
[IV] Studying the SET/RESET behavior from the energy point of view was an idea that emerged last year. Such study can reveal the energy-efficiency of this new device technology, which is required for modern memory and computing applications. Moreover, energy is a magnitude that can be physically explained; for instance, every conductive channel requires a minimum amount of energy to be created/dissolved. Therefore, inspired by previous studies carried out on unipolar memristors by our collaborators in Universidad Autónoma de Barcelona, Spain, we proceeded to compute the average energy cost of resistive switching (RS) events in Knowm memristors. This allowed us to figure out the quantitative effect of the injected energy on the resistive state of the devices. We showed that the average energy required in the SET/RESET generally demonstrates low variability and it is independent of the biasing conditions. Based on our analysis presented in [3], we proposed the “resistance change per energy unit” (dR/dE) as the first universal performance parameter, which could be extracted for any RS device family to be used for comparison purposes and/or in device modeling tasks. Our results showed that while the dR/dt change rate depends on the actual resistance of the device (i.e. the lower the resistance, the slower the switching rate), for any given R the average dR/dE is kept almost the same. Most importantly, we confirmed the observed trends in data taken not only using Knowm memristors but also other totally different bipolar RS devices (different in terms of material structure and switching mechanism). Furthermore, we devised a simplified mathematical model for energy consumption, to the best of our knowledge being the first of its kind, attributing the energy delivered to the memristor during the switching process to Joule heating and to a critical energy component, which is required to induce the resistance change. We achieved a surprisingly good quantitative matching between our model and the experimental data from Knowm devices. Everything considered, we believe that our experimental results confirm that the proposed dR/dE is a comprehensive magnitude, suitable not only for the behavioral analysis of the analog operation of memristors, but also to be used as a universal performance parameter capable of characterizing quantitatively different analog RS device families.
[3] J. Gomez, I. Vourkas, A. Abusleme, R. Rodríguez, J. Martin-Martinez, M. Nafria, and A. Rubio, “Exploring the “Resistance Change per Energy Unit” as Universal Performance Parameter for Resistive Switching Devices”, Elsevier Solid State Electronics., vol. 165, no. 107748, March 2020
Our results showed that while the dR/dt change rate depends on the actual resistance of the device (i.e. the lower the resistance, the slower the switching rate), for any given R the average dR/dE is kept almost the same.
[AN] What are your future plans in the field?
[IV] I am really happy to see that more and more students are gradually getting involved in memristor-related research and development tasks in my group, and see that we are able to get to clever solutions at circuit level. My research is funded by national research grants and by the Advanced Center for Electrical and Electronic Engineering (AC3E) in our University, where I am Associate Researcher. Nevertheless, I believe we can get to even better results combining the expertise of groups working in different disciplines and at different levels of abstraction, from device physics to applications. Actually there are a couple of short-term projects I am working on, while preparing the field to be able to work with large arrays of memristors, such as the crossbar arrays available in chips that Knowm released. I am confident that soon we will have some interesting results to share with you.
[AN] Where do you see the field going in the next 5 to 10 years?
[IV] Well, technology will not cease to evolve, neither will the memristor devices. During the last 10 years the number of researchers from different disciplines working/collaborating in this field has increased significantly and this interaction will certainly enable real implementation of applications for memristors, as well as contribute to better understanding of their behavior. Probably, the fact that still there has been nothing “big” that reached to the market, might be discouraging for the most conservative ones, even though there were “big” announcements in the last few years. But we work and learn more about this technology day after day. Devices have their imperfections, such as the cycling variability, but still it depends on us, the engineers, whether we can make use of them, or simply wait for the perfect memristor (which could take forever). Nevertheless, we have evidenced important steps towards commercial establishment of memristors; for instance, the fact that anybody can access real discreet memristor devices thanks to Knowm Inc. initiative. Moreover, the number of companies around the world, relevant to this field, is steadily increasing. For example, Crossbar Inc., Memryx, and Memcomputing Inc. in USA, are just a few examples, as well as Arc Instruments in UK, for ad-hoc instrumentation solutions for memristor technology. We should also bear in mind that the first few small-scale nonvolatile resistive RAM (ReRAM) chips have already been announced. The introduction of faster and more energy-efficient ReRAM memories will certainly boost machine learning and AI applications. Everything considered, nowadays, it is more than clear that there are memristor-based circuit solutions that outperform conventional circuits for several tasks including storage, computing, and the combination of two in computational memories. I am confident that within the next 10 years, with patience and perseverance, we will achieve/experience something really revolutionary!
Everything considered, nowadays, it is more than clear that there are memristor-based circuit solutions that outperform conventional circuits for several tasks including storage, computing, and the combination of two in computational memories. I am confident that within the next 10 years, with patience and perseverance, we will achieve/experience something really revolutionary!
[AN] What EDA tools, especially open-source, do you use?
[IV] While exploring memristor behavior, I usually created my own code for simulation using high level mathematical tools such as Matlab or any programming language. The same applied for the simulation of memristor-only circuits. You do not need a circuit simulator to do that, if you know how to apply Kirchoff´s Laws. This is the same exercise I put to my students, and helps them a lot to understand memristor models and collective memristor behavior. There is a simulator of the Crossbar topology called “XbarSim” which we created in JAVA while I was still a PhD student, mostly for educational purposes. I have shared it many times (upon request) with students and researchers from all over the world. Once true circuit simulations are required, combining memristors with other electronic devices, I usually prefer LTSPICE which is freeware. Starting directly with SPICE simulations might sometimes cause frustration, especially to those who are not familiar with netlists and circuit-level description of a device model. But if you have simulated your model before translating it into a SPICE netlist, you know what to expect so debugging is easier. As far as commercial EDA tools are concerned, in my VLSI course I currently use uWind (microwind) for layout design, which was developed to deliver a better learning experience. Soon I have plans to move to the Synopsys tools, given that here in Chile we have an R&D department of Synopsys and the guys there are always willing to help and promote microelectronic circuit design projects in Universities.
[AN] What research papers or background literature would you recommend any new memristor student read?
[IV] Well, there are a lot of great works out there. But regardless of being great or not, I believe the most important thing is being easy to follow and understand. Assuming that memristor models are the first thing to study and understand, I usually recommend starting from the HP linear model and its SPICE description found in [1] and the simple threshold-type model with readily available netlists found in [2].
[1] Z. Biolek, D. Biolek, and V. Biolkova, “SPICE model of memristor with nonlinear dopant drift,” Radioengineering, vol. 18, no. 2, pp. 210–214, 2009
[2] Y. Pershin and M. Di Ventra “SPICE Model of Memristive Devices with Threshold,” Radioengineering, vol. 22, no. 2, pp. 485–489, 2013
By coincidence, these are papers published in the same journal. These models have just a few equations, few variables whose role is straightforward to understand, and most importantly, concern fundamentally different device behavior. On one hand we have a current-controlled model [1] without thresholds, whereas on the other hand we have a voltage-controlled model with thresholds [2]. So, they help to understand better some important notions, including the role of window functions in memristor modeling, and the fact that different models will lead to different simulation results. Afterwards, one is ready to move to more complex/complete models; a useful review can be found in [3], although there are more models out there published more recently.
[3] C. Yakopcic, T. M. Taha, G. Subramanyam, and R. E. Pino, “Memristor SPICE Modeling,” in: Kozma R., Pino R. E., Pazienza G. E. (Eds.) Advances in Neuromorphic Memristor Science and Applications, Springer Series in Cognitive and Neural Systems, vol 4. Springer, Dordrecht, 2012, pp. 211-244
As far as applications are concerned, I usually recommend reading a couple of papers that I find inspiring and still easy to follow. For instance, the potential of memristor networks to rapidly solve mazes and to compute shortest paths in complex graphs mapped onto networks of memristors; a recent publication is found in [4], although it was Max Di Ventra and Yuriy Pershin who were the first to present such fantastic ideas. Their papers from 2011-2013 really inspired me to work in this topic.
[4] A. Mizrahi, T. Marsh, B. Hoskins, and M. D. Stiles, ‘‘Scalable Method to Find the Shortest Path in a Graph with Circuits of Memristors,’’ Phys. Rev. Applied, vol. 10, no. 064035, 14 December 2018
In the same context of complicated computing tasks, such as solving a linear system or solving a differential equation which generally requires a large number of computing steps and an extensive use of memory, in-memory computing with ReRAM provides a promising solution, owing to the compact multi-level data storage and the physical computation realized in memory. For instance, it was recently shown in [5], [6] that matrix-vector multiplication can be performed with O(1) complexity using a crossbar resistive memory where the matrix values were mapped linearly to the conductance of the memristive cross-points, thus proving a significant speed-up over the polynomial time complexity of classical digital computers.
[5] A. Sebastian, et al., “Tutorial: Brain-inspired computing using phase-change memory devices,” Journal of Applied Physics, vol. 124, no. 11, pp. 111101, 2018
[6] Z. Sun, et al., “Solving matrix equations in one step with cross-point resistive arrays,” PNAS, vol. 116, no. 10, pp. 4123-4128, 2019
There are several such technical papers out there that demonstrate the great potential of memristors, especially those with experimental verification. But instead of listing all possible applications and their respected recommended papers, I’d suggest taking a look at a couple of papers that highlight the fact that memristors could really upgrade conventional circuits, converting them from static to programmable and/or adaptive just by replacing a key-role resistor with a memristor. For instance, I usually recommend reading [7] where some analog circuits are presented, with their memristive version. However, memristors are “ubiquitous” and can be actually useful in several different tasks occurring on-chip: from multi-level nonvolatile storage and in-memory computing, to data converters in the interface circuits, clock generation and even local signal transmission. In this sense, we prepared a review of such applications in a book chapter found in [8].
[7] Y. V. Pershin, and M. Di Ventra, “Practical Approach to Programmable Analog Circuits with Memristors,” IEEE Trans. Circuits and Systems-I: Reg. Papers, Vol. 57, No. 8, pp. 1857- 1864, 2010
[8] I. Vourkas, M. Escudero, G. Ch. Sirakoulis, and A. Rubio, “Ubiquitous memristors in multi-level memory, in-memory computing, data converters, clock generation and signal transmission,” in: P. Dimistrakis, I. Valov (Eds.) “Metal Oxides for Non-volatile Memory: Materials, Technology and Applications (1st Ed.),” Elsevier, 2020
Subscribe To Our Newsletter
Join our low volume mailing list to receive the latest news and updates from our team.