Neuromorphic Computing Symposium 2017 – Architectures, Models and Applications
In part a response to the Nanotechnology-Inspired Grand Challenge for Future Computing initiative, some of the key players in the neuromorphic and neuromemristive space gathered at the Crowne Plaza in Knoxville, Tennessee to present new research, develop collaborations, and provide a forum to publish work in this area. The symposium was heavily attended by University of Tennessee and Oakridge Laboratory researchers, as expected, but also drew participants from around the country. Knowm Inc was lucky to have the opportunity to sponsor the dinner with Duke university and provide a great ice-breaking atmosphere of free-flowing wine and great food to encourage idea exchange and future collaborations. If the USA is going to lead this race, it will require a great deal of teamwork.
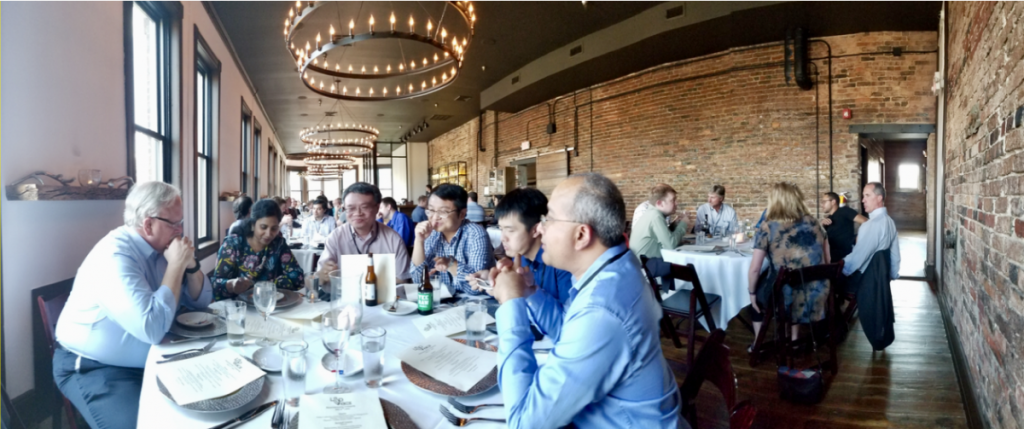
Neuromorphic Symposium Dinner Sponsored by Knowm Inc and Duke University, Knoxville Tennessee, July 2017
Stan Williams – HP
Stan Williams gave a very strong presentation and made great contributions to the discussion over multiple days. Williams reviewed HP’s continued involvement in the development of memristive technology and gave a rallying cry for the Gen-Z Consortium, which is “an open systems interconnect designed to provide memory semantic access to data and devices via direct-attached, switched or fabric topologies.” Williams presented impressive results from HP collaborators at UMass Amherst (which we saw for the first time at the ECE Conference), which included (1) programmable memristive crossbars, which they are demonstrating for use in image processing applications as well as (2) what they call “competitive computing” where they have demonstrated simple learning circuits. While the similarity to AHaH Computing has not escaped our attention, we are excited that others are converging on the same ideas.
Catherine Schuman
Catherine Schuman was out in force, presenting multiple times and leading a session. Of particular interest was (1) her absolutely massive literature review of the neuromorphic computing field, which will be arriving as a review paper shortly and (2) her group’s results showing their genetic algorithms actually beat convolutional neural networks on a non-trivial benchmark task.
Dhireesha Kudithipudi
Dhireesha Kudithipudi presented results from a systems-level integration of deep convolutional networks and reservoirs for transfer learning, showing results that bested more traditional ML techniques and provides a path toward efficient hardware implementations for hand-held devices.
Jack Kendall
Jack Kendall presented some refreshing ‘out of the crossbar’ thinking from his start-up company Rain Neuromorphics, an offshoot of work at University of Florida. The core idea is to take advantage of the oxides that form over nanowires laid down in a ‘rats nest’ to form long-range memristive connections. By taping this tangle of nanowires with electrode ‘pilars’, we have a new type of all-to-all connective not conditioned on the typical cross-bar arrangement and potentially offering new possibilities. The group has shown (theoretically) how such a network could implement back-propagation of error.
Alex Nugent
Knowm CEO Alex Nugent presented recent work on a new form of compositional (deep) learning not based on the backprop algorithm that can more easily be mapped to neuromemristive processors such as kT-RAM as well as presented a poster on the open-source Memristor Discovery platform.
Beyond CMOS: From Devices to Systems
The Beyond CMOS: From Devices to Systems conference on June 5-6, 2017 took place in Meyer Building (EE) Technion, Haifa, Israel. Knowm’s Tim Molter attended all the memristor talks and presented a poster and demonstration of Knowm’s Memristor Discovery to interested parties. All in all, there was a lot of excitement about using memristors to go “beyond Moore’s law and CMOS”, and from Knowm’s point of view the industry is continuing to converge on the idea that using memristor pairs (instead of single memristors) for synapses is the way forward. Many new device types were presented and well as some rudimentary machine learning demos and STDP behavior.
Tim’s poster can be downloaded here: Beyond_CMOS_2017_Knowm_Tim_Molter_Poster.pdf.
Giovanni De Micheli, EPFL – Emerging Technologies for Computing
Giovanni De Micheli is Professor and Director of the Institute of Electrical Engineering and of the Integrated Systems Centre at EPF Lausanne, Switzerland. He is program leader of the Nano-Tera.ch program.
- Tri-gate transistors – lower leakage, faster
- Box (buried oxide) transistors
- 7 to 5 nm nodes
- silicon nanowire transistor
- Carbon nanotube transistor
- Oxide resistive RAMs TaOx
- Switch from von Neumann arch.
Onur Mutlu, ETH Zurich/CMU – Rethinking Memory System Design (and the Computing Platforms We Design Around It)
- DRAM “row hammer” security problem in dense arrays – flipping bits really fast before a DRAM cap refresh occurs affects adjacent rows.
- Manufacturers had to release patches to fix this security hole
- Need a paradigm shift to eleiminate data movement (von Neumann architecture) – more memory-centric
- In-memory page copying – eliminate round trip to CPU.
- Orders of magnitude improvements for new memory-centric architectures
Engin Ipek, University of Rochester – Memristive Accelerators for Data Intensive Computing: From Machine Learning to High-Performance Linear Algebra
- In-Situ Memristive Accelerators
- Combinatorial optimization
- Analog dot product computation with memristors
- First time we saw Htree-Controller-RAM on a slide
Michael Kozicki, Arizona State University – Applications of Cu-SiO2 Devices in Electronic Systems
- Cu-silica-Pt devices – low temp process
- Schottky diode produced by Cu electrode for eliminating cross talk
- 10 pA programming current – took a week to program though.
- nice incremental response – relatively linear and symmetric – for neuromorphics
- CBRAM by Adesto
- 3d cross point – coming soon
Thomas Mikolajick, Namlab and TU Dresden – Nonvolatile Memories based on Ferroelectricity in Hafnium Oxide and Zirconium Oxide
- Ferroelectric memory – for niche applications
- hysteresis observed in HfOx doped ferroelectrics
- HfOx Ferroelectric memory potentially optimal for new memory
- Fe-FET for STDP operated as synapse
Eli Yablonovitch, UC Berkeley – The Scientific Challenge of Replacing the Transistor with a Lower Voltage Device
- Landauer’s limit says all electronics are operating 10,000 times more energy budgets than they need to.
- Canter for Energy Efficient Electronics Science
- At the nanoscale we need atomic scale precision
Michal Lipson, Columbia University – Next Generation Silicon Photonics
- Data Center Crisis – Microsoft dropping their datacenters in the ocean
- Power dominated by the communication from RAM
- CMOS ring waveguides of different diameters capture different wavelengths, all other wavelengths pass by.
- Inject a voltage signal in the waveguide and the index of refraction changes
Ioannis Vourkas, PCUC – Studying the Resistive Switching Behavior from the Energy Point of View
- Stochastic Nature of Resistive Switch
- Energy require to switch state not dependent on applied signal rise time, but the energy required is different
- Knowm memristor showing hysteresis response
Mircea Stan, University of Virginia – Back to the Future: Digital Circuit Design in the FinFET Era
- FinFETs make CMOS doable at 0.2V Vdd
- Independent-gated finFET can act as a logic gate
- FinFETs allowing continuation of Moore’s Law
- The fins are getting closer together AND higher
- Intel calls this hyperscaling
- Aspect ratio of 25 demoed in lab
Pierre-Emmanuel Gaillardon, University of Utah – Towards Lower-Power High-Performance FPGAs with RRAM-Based Routing
- RRAM – non-volatile storage, radiation tolerant, engineered properties
- FPGAs inefficient architectures – routing and routing multiplexors
- Replace switches (transmission gates) in MUX with RRAM
- In RAM 4T1R can actually give you more density than 2T1R when comparing power density
Edwin Kan, Cornell – Hybrid Ferroelectrics-CMOS: Devices and Circuits
Stuart Parkin, Max Planck Institute – Chiral Spin-Orbitronics
- MTJ – magnetic tunneling junction
- 2016 MRAM now commercially available
- $1B in funds to make it possible
- 3d racetrack memory increases memory density by 100x
Leon Chua, UC Berkeley – 10 Things You Didn’t Know About Memristors
- The only valid DC-cureve for a memristor is a single point at the (0,0) origin
- egg white can be used as a memritor
- Not all memristors are non-volatile
- Synapses are memristors
- Axons are made of memristors
- Some memristor circuits have Hamiltonians
Damien Querlioz, Paris-Sud University – Neuromorphic Computing with Spintronics
- ST-MRAM is reaching maturity
- using magnetic tunnel junctions as synapses – The MJTs are binary as far as I can tell, so not like synapses at all.
- cross bar architecture where MTJs are at each cross. Simplified STDP for signals.
- Detecting cars in multiple lanes, but this is such a trivial demo, I don’t believe M.L is even necessary – completely linearly seperable.
Jennifer Rupp, MIT – Defect Engineering for Oxide-Memristors
- Adesto maviq 512 kb memristor RAM
- Memristor pairs as synapses – addressing each memristor individually and as a pair
Sabina Spiga, CNR-IMM – Tailoring RRAM for Neuromorphic Computing
- HfOxRRAM – Hafium
- Analog synapses – incremental response to pulses.
- Their memristors look like good condidates for kT-RAM
- MNIST benchmark on a real chip with memritsors connected to separate CMOS chip via pads 65% performance
Giacomo Indiveri, ETH Zurich – Real-Time On-Line Learning and Computing in Event-Based Neuromorphic Memristive Architectures
- Speed not a requirement for brains
- Bees – 10^-15 J/spike, 960,000 neurons, 1 mm^3 volume, 1 mg weight
- asynchronous self-clocked circuits, non von Neumann acrchs., co-localized memory and computing elements, new programming pardigms.
- 28 nm FD_SOI multi-core Neuromorphic processor
- Shows many simulated M.L capabilities: robotic control, Comb. Opt.
- Attractor networks to do computation
Abu Sebastian, IBM – Phase-Change Memory: Applications in Neuromorphic Computing
- Watson – 2880 core, 80 kW of power, 20 tons air conditioning cooling capacity
- NOt interested in exact results, in place computing provide approximations that are good enough
- PCM core for MNIST solver neural network
- spiking neural networks – what re the killer apps is the #1 challenge
- IBM TrueNorth – no in-situ learning – PCM neuron can help here
- 64k PCM core built at IBM
Hai Li, Duke University – Emerging RRAM Technology Enabled Cognitive Computing Systems: Challenges and Opportunities
- Memristor crossbar to mimic neural network
- “Brain-Ste-in-a-box”
- Using two cross bar arrays –
- level based design use digital to analog voltage applied to synapse inputs
- Working with HP labs
Massimiliano Di Ventra, UCSD – Memcomputing: a Brain-Inspired Topological Computing Paradigm
- Memcomputing Inc. Fabio Travera, John Beane – brain inspired topological computing
- NP-complete problems – standard alg.=2^N explosion, memcomputing=N^4
- NP hard problems in polinomial time – 2 orders of magnitude better than competitors in competitions
- random initial conditions evolve over time and converge on the solution
- Like quantum computers but ready to be built today – “classical objects”
- Seems like smoke and mirrors to me in the way he presented it. No attempt to explain it to a layman.
EMN Memristive Switching & Networks
The EMN Memristive Switching & Networks on August 14-18, 2017 took place in Milan Italy. The memristor session was relatively small, but a lot of exciting research and advancements was presented.
Martin Klimo – University of Zilina, Slovakia – Memristive Implementation of Fuzzy Logic and Neural Networks
- Von Neumann architecture good for arithmetic/algorithmic computing.
- Cognitive computing requires a different kind of hardware.
- Both types of architecture will cooperate.
- Fuzzy logic system uses nearest neighbor pattern matching calculating distances from the input data to the feature library.
- The next challenge is to reduce the number of memristsors needed to hold all the patterns.
Masayoshi Higuchi – National Institute for Materials Science, Japan – Metallo supramolecule polymers as memristor
- Metallo supramolecule polymers as memristor.
- The color of the material can be changed by applying a 1V signal to it (electrochromism).
- Using different metals creates different color ranges.
- Color state remains after removing voltage signal.
- The material exhibits a hysteresis loop like a memristor.
Mehdi Anwar – University of Connecticut, USA – Memristors: Material, Device, and Systems
- Creation of Zinc Oxide memristors as nanowires.
- CMOS compatible. MOCVD growth. ZnO memristor (horizontal nano-cone) LRS=189 Ohm, HRS = 129 kOhm.
- Filamentary dictated transport.
- Most of the work they do is on secure electronics: physical unclonable functions (PUF).
Gennady N. Panin – Russian Academy of Sciences, Russia – Photomemristive Systems Based on Two-Dimensional Crystals
- Memory based on graphene is bio-compatible and can self assemble.
- Current < 1 nA, switching voltage < 0.7V. I-V characteristics dependent on light excitation.
Ken Saito – Nihon University, Japan – Artificial Insect Equipped with Hardware Neural Networks
- Miniature nano robots will be used for medical applications inside human bodies.
- Body parts are all made of silicon wafer material. Locomotion is like an ant walks: alternating tripod gait.
- Temperature sensitive wire coils expand and shrink.
- Future work will integrate the power source, sensory and controlling system on the robot as well.
- Electrostatic inchworm motors.
Kouichi Takase – Nihon University, Japan – Switching property of nanowire based ReRAM
- Trying to figure out why the switching voltage of oxide memristors as such a large distribution.
- He thinks is the many possible filamentary connections that form during each cycle.
- His solution was to first coat the wafer surface with vertically-standing 500 nm nano wires densely packed.
- This produced good switching windows (no overlap between Von and Voff).
- 5 minutes of oxidation additionally improves the switching voltage distribution (narrower).
Josep L. Rosselló – Balearic Islands University, Spain – Hardware Neural Network: Theory and Applications
- General talk on STDP-based memristor crossbar neural networks.
- Memristors provides the highest performance but has the lowest flexibility and endurance.
- Stochastic spiking neuron (SSN) was implemented in FPGA.
- Successful results on handwritten digit benchmarks.
Nan Du – University of Technology Chemnitz, Germany – Resistive Switching dynamics in memristive BiFeO3 (BFO) Device
- Analog bipolar resistive switching device.
- Electro-forming free.
- 10000+ cycle endurance perfect.
- Set/reset is +/- 8 V.
- All 16 logic functions realized with bilayer memristor.
- STDP functions demonstrated.
vReally good looking bidirectional incremental behavior.
Alex Nugent – Knowm Inc.,USA – Memristors to Compositional Learning
- Introduction to Knowm’s Memristor symbol
- Define life as self-organizing mechanism to harness free energy and intelligence as the ability to learn
- The future of computing is asking for synapses – lot of synapses
- Introduced the kT-Synapse – in nature and as a memristor differential pair and showed many examples of other researchers converging on the idea as well.
- Introduced compositional learning with kT-RAM
- Talked about Knowm’s full stack AI framework from memristors to deep learning
- Introduce Knowm memristors and Memristor Discovery
2017 USENIX Vail Computer Elements Workshop
The USENIX Vail Computer Elements Workshop is a unique four day workshop that has been around for 47 years serving leading architects of the computer industry. This intentionally small workshop is intended to allow a lively interaction between the participants and the speakers.
The venue was informal, lively and engaging and certainly unlike most conference we have been to.
Subscribe To Our Newsletter
Join our low volume mailing list to receive the latest news and updates from our team.