AHaH Computing, Thermodynamic Computing, Competitive Computing, Memristive Dot-product Engines, Dual Memristor Cross bars – are these all different names or variation of the same thing? All are gaining the attention of the academic and commercial research community, and for perhaps the first time ever, we felt a coherence and alignment of views that this upcoming technology is what will drive the next quantum leap in computation. The 2017 Energy Consequences of Information (ECI) conference was a workshop dedicated to 1) understanding the state‐of‐the‐art in energy optimization and utilization for information processing, including computation, storage, and transfer, 2) surveying current technologies and articulate areas requiring research and development, 3) leveraging community expertise in emerging technology to brainstorm new research pathways, 4) developing a rational roadmap for fundamental research and technology development, and 5) understanding the potential roles of government, industry, and academia.
The following is a summary of the talks that we found the most relevant, impressive and inspiring. As we have been developing theories and technology in this specific area for a very long time, it was great to see so much relevant work and talented people elucidate their work regarding the ‘energy consequences of information’.
Thermodynamic Computing
Todd Hylton, an adjunct professor at UCSD and friend of Knowm Inc. CEO Alex from former days working together on DARPA’s Synapse program, gave the session keynote titled “Thermodynamics and the Future of Computing”. In his presentation, he presented his ideas surrounding “Thermodynamic Computing”, whereby a hardware architecture would be set up containing loosely coupled nodes in a network, some hard-coded to represent some pre-determined function, and others allowed to evolve into their own unassisted state or function over time. The raw data from the environment would flow into the system and the nodes would evolve automatically to optimize a desired high-level objective. A thermodynamically evolving intelligent system like this would have the potential to operate near thermodynamic limits of efficiency. Learn more about Thermodynamic Computing.
Competitive Computing
J. Joshua Yang, a professor at University of Massachusetts and sponsored by HP, DARPA, IARPA and AFRL presented his latest research titled: “Experimental demonstration of analog computing and neuromorphic computing with memristor crossbar arrays”. There were two things presented that were really impressive to us. First off, the oxide-based memristive devices they developed exhibit phenomenal behavioral characteristics amenable to neuromorphic computing. The positive and negative incremental response to voltage pulses ranging between 0.5 V and 1.0 V were repeatable and consistent. One particular demonstration of repeated pulses showing 64 discrete states was especially nice to see. With these devices they were able to create a dual cross bar array of 64X128 cells, write a desired conductance and read out the conductance of the devices individually. They even encoded the AFRL logo onto the dual cross bar array, which they animated into a video. While there were a few glitches, this is a major step forward in cross bar technology. Hats off to those efforts! Secondly, something that we’ve appreciated for a long time now and which forms the basis of AHaH computing, he showed the importance of using memristor pairs, not just individual memristors, to represent the synaptic weight. He mentioned the term “Competitive Computing” for this reason. Only when using a pair of memristors can you have a weight that provides both a magnitude and a state – both essential in doing synaptic integration and setting up competitive dissipative pathways for self-evolving thermodynamically-driven AI. This work reminded us of a previous demonstration of this by Truong et. al, although while they did actually demonstrate pattern recognition with it, it was only a 3x3x2 dual crossbar and not a 64x64x2 crossbar.
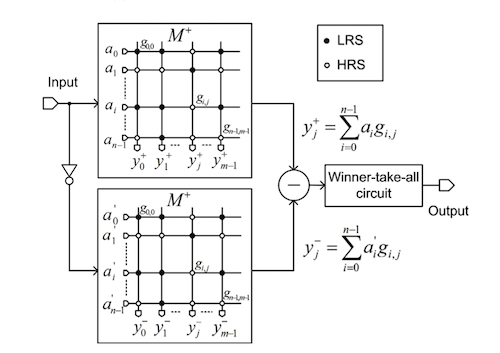
Dual Crossbar
More Dual Crossbars
Dimitri Strukov, associate professor at UC Santa Barbara, presented his work with a presentation titled “Application of UPSIDE Technology”. In his talk he provided quantitative evidence that memristor-based neural processing units targeting machine learning applications will provide at least a 10,000x power efficiency advantage over typical von Neumann architectures such as CPUs, GPUs, FPGAs, and even specialized deep learning digital specialized chips such as IBM’s True North. He then showed simulation results of a dual crossbar array successfully performing the MNIST classification benchmark at primary performance metrics congruent with standard machine learning linear perceptron results. While it was just a simulation, he does have previous impressive work (Training and operation of an integrated neuromorphic network based on metal-oxide memristors) showing physical dual crossbars doing pattern recognition and classification on a set of 3×3 binary image patterns. Again, what this demonstrates is the necessity of differential conductance weights.
Honorable Mentions
Three additional presentations stood out, and while they didn’t exactly relate to thermodynamically-driven neuromorphics, the concepts are definitely key to where the field of computing is heading.
Paul Armijo, director at GSI Technology, presented his Associative Processing Unit (APU) that changes the concept of computing from serial data processing—where data is moved back and forth between the processor and memory—to massive parallel data processing, compute, and search in-place directly in the memory array. This in-place associative computing technology removes the bottleneck at the I/O between the processor and memory. Data is accessed by content and processed directly in place in the memory array without having to cross the I/O. The result is an orders of magnitude performance-over-power ratio improvement compared to conventional methods that use CPU and GPGPU (General Purpose GPU) along with DRAM. We think that this technology has the potential to play a significant role in accelerating raw data encoding as well as CNN operations such as convolutions and pooling operations. While this architecture doesn’t reduce the distance between processor and memory to exactly zero like in brains, it does get extremely close, and it will probably play an important role in future analog+digital computing systems.
Natesh Ganesh from the University of Massachusetts, Amherst presented a talk titled “Fundamental Limits on Energy Dissipation in Neuromorphic Computing”. He defines the “minimal dissipation hypothesis” as open physical systems with constraints on their finite complexity, that dissipate minimally when driven by external fields, will necessarily exhibit learning and inference dynamics. While we would state that physical systems appear to maximize free-energy dissipation and entropy (under whatever constraints), we’re describing both sides of the same coin. We’re happy to see additional excitement and energy being poured into this new and exciting branch of fundamental physics!
Garrett Kenyon from LANL gave a presentation about sparse encoding video streams using dual binocular cameras. This impressive work used a neat trick, which biology may employ given the presence of two eyes on most animals, whereby convolutional filters or kernels are designed to pull out information from the differences between the two cameras aiming at the same scene. This differential perspective allows the system to leverage additional depth information, which normally would not be present. We also appreciate his shout out to Alex’s early work on some of the foundational concepts that lead to DAPRPA’s Synapse program and it’s successor UPSIDE. See Unsupervised Adaptation to Improve Fault Tolerance of Neural Network Classifiers and Reliable computing with unreliable components: Using separable environments to stabilize long-term information storage;
Convergence?
Were the objectives of the conference met? There were representatives from academia, large tech companies, start-ups and governmental funding agencies, each presenting their own perspectives of what’s important in low energy computing. As the conference came to a close and the organizers gave their closing remarks, it did feel like there was some consensus in the group. While there was a desire for more follow up on Landauer’s limit, adiabatic computing and digital system optimizations, the over-arching consensus was that Moore’s Law is running straight towards a brick wall, and it is necessary to rethink from the ground up a new type of computing where the adaptive power problem is eliminated by thermodynamically-evolving adaptive electronics. While a small handful of presenters including Natesh, Todd, Garrett and Alex explicitly made a call for this, it was interesting to see that others such as Dimitri and Yang, with their dual crossbars and memristor-pair synapses, a.k.a. kT-Synapses, are on a direct path to it whether they realize it or not at the moment.
For our perspective on the state of computation and the inevitable future direction it will move in, here is Knowm Inc’s CEO, Alex Nugent, presenting at the ECI conference.
Subscribe To Our Newsletter
Join our low volume mailing list to receive the latest news and updates from our team.